Oz im ML: A Comprehensive Guide to Transforming Healthcare with Machine Learning
Introduction
Machine learning (ML) has emerged as a transformative force in the healthcare industry, offering unprecedented opportunities to improve patient outcomes, optimize operations, and empower clinicians. This comprehensive guide delves into the vast landscape of Oz im ML, exploring its applications, benefits, challenges, and strategies for successful implementation.
The Power of ML in Healthcare
ML algorithms can sift through vast volumes of healthcare data to identify patterns, predict outcomes, and make informed decisions. This enables healthcare providers to:
-
Improve patient care: Diagnose diseases earlier, tailor treatments, and predict patient responses.
-
Optimize operations: Reduce costs, improve efficiency, and streamline workflows.
-
Empower clinicians: Provide real-time insights, assist in decision-making, and free up time to focus on patient care.
Applications of ML in Healthcare
ML has found numerous applications in healthcare, including:

-
Disease diagnosis and prediction: Identifying and predicting conditions such as cancer, heart disease, and diabetes.
-
Treatment planning: Tailoring treatments to individual patients based on their medical history and genetic profile.
-
Drug discovery and development: Accelerating drug development and improving drug efficacy.
-
Medical image analysis: Detecting abnormalities and diagnosing diseases from medical images.
-
Clinical decision support: Assisting clinicians in diagnosing and managing patient conditions.
Benefits of Oz im ML
-
Improved patient outcomes: Earlier diagnosis, more effective treatments, and reduced complications.
-
Cost reduction: Streamlined operations, reduced hospital stays, and optimized drug regimens.
-
Increased efficiency: Automated tasks, improved workflow, and better resource allocation.
-
Empowered clinicians: Real-time insights, improved decision-making, and enhanced patient engagement.
Challenges of Oz im ML
-
Data quality and availability: Ensuring data is accurate, complete, and accessible.
-
Algorithm bias and interpretability: Addressing potential biases in ML algorithms and ensuring their interpretability to clinicians.
-
Ethical considerations: Navigating issues related to privacy, data security, and algorithmic fairness.
-
Lack of skilled workforce: Addressing the shortage of qualified professionals with expertise in ML and healthcare.
Strategies for Successful Oz im ML Implementation
-
Define clear goals and objectives: Determine how ML will support specific healthcare objectives.
-
Invest in data quality and infrastructure: Ensure access to high-quality data and robust technical infrastructure.
-
Build a multidisciplinary team: Foster collaboration between clinicians, data scientists, and IT professionals.
-
Pilot and evaluate projects: Start with small-scale projects, evaluate results, and iterate to improve.
-
Promote clinical adoption: Engage clinicians throughout the implementation process to ensure acceptance and adoption.
Tips and Tricks for Effective Oz im ML
-
Use validated algorithms: Choose ML algorithms that have been validated for healthcare applications.
-
Consider patient context: Incorporate patient-specific factors into ML models to improve accuracy.
-
Monitor and update models: Regularly evaluate and update ML models to ensure they remain effective over time.
-
Seek regulatory compliance: Adhere to applicable regulatory guidelines for data privacy, security, and ethical use of ML.
Step-by-Step Approach to Oz im ML Implementation
- Define goals and objectives.
- Gather and prepare data.
- Choose and train ML algorithms.
- Deploy and evaluate ML models.
- Monitor and update models.
Stories and Lessons Learned
Story 1: A leading hospital used ML to predict sepsis early, reducing sepsis-related mortality by 30%.
* Lesson: ML can significantly improve patient outcomes by identifying high-risk conditions early.
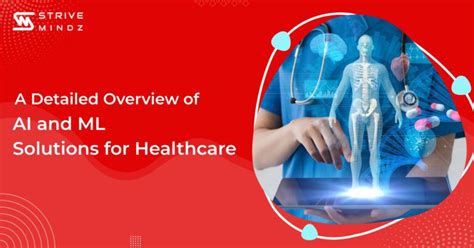
Story 2: A healthcare provider implemented ML-based automated prescription filling, reducing errors by 50%.
* Lesson: ML can streamline operations and improve patient safety by automating tasks prone to human error.
Story 3: A pharmaceutical company used ML to accelerate drug discovery, reducing the time and cost of bringing new drugs to market by 25%.
* Lesson: ML can revolutionize drug development by identifying new targets and improving drug efficacy.
Effective Strategies
-
Adopt hybrid approaches: Combine ML with other technologies such as artificial intelligence (AI) and natural language processing (NLP) to enhance capabilities.
-
Foster collaboration: Encourage partnerships between healthcare institutions, academia, and technology companies to drive innovation.
-
Invest in education and training: Provide training programs for clinicians and healthcare professionals to build a skilled workforce in ML.
-
Promote data sharing: Establish mechanisms for secure and responsible sharing of healthcare data to facilitate research and development.
Conclusion
Oz im ML is a transformative technology that holds immense potential to improve healthcare outcomes, optimize operations, and empower clinicians. By embracing ML and adopting effective implementation strategies, healthcare organizations can harness the power of data and technology to revolutionize the delivery of care. As the field of ML continues to evolve, we can expect even greater advancements and breakthroughs that will redefine the healthcare landscape.
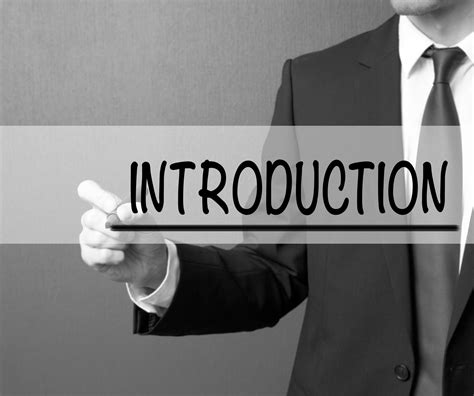